**refer to Drott article and Laplante**
Dr. McGraw, Department of Music at the University of Richmond, ethnomusicologist and successful publisher, joins us in an interview concerning his personal experience and expertise regarding recommender systems in the music industry, how a user might best find a new song, and to understand the complexity of music technology and its overall effects on users. He posits the goal of a recommender system is to provide users with a product– a song, movie, household item, etc. –that they didn’t realize they liked based on the choices they have made thus far. Due to the fact that things change so frequently and technology is constantly evolving, Dr. McGraw hasn’t found one platform that has been consistently successful. However, his knowledge and critiques might be able to point us in a direction that leads us closer to learning how to increase the success rate of recommender systems and discover methods for ourselves and other listeners.
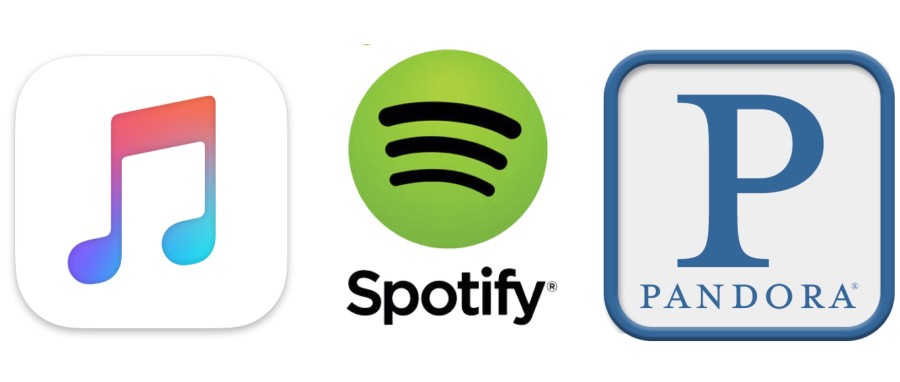
(Jansen 2015)
A key question to ask ourselves is: what did you use and when? An avid Pandora user when it first came out, Dr. McGraw has then since switched to Spotify, appreciating its feature to view an entire discography of a particular artist immediately and be able to do due diligence with a genre. Yet, that’s not a recommender system despite its conveniences and perks. Most of these applications have basic and premium account options that allow users to skip ads, and increase their experience as a listener but Dr. McGraw was and still is to this day getting recommendations that he didn’t agree with and that simply put are terrible.
At the inception of music recommendations, there were a lot of Artificial Intelligence tools or machine learning tools like manual tagging or music grad students generating metadata on Excel sheets, explains Dr. McGraw. However, concepts that create suggestions off of aspects like BPM (beats per minute) don’t work for all genres, especially classical music. Additionally, “structural elements like sections or …. texture, also known as timbre” may be used as a descriptor yet not completely represent the tracks as a whole (Horsburgh, Craw, Massie, 28). The AI embeds “all kinds of assumptions about Western classics… assuming there’s one tempo, one key, one form, and song structure”, says Dr. McGraw. It gets interesting when AI was given more freedom to find features on its own, since it may find features that as humans, we would not have identified as similarities. As we draw away from human-guided machine learning, and more into this space of non-supervised learning, that’s when we start to receive those “off-the-wall” recommendations that resemble the radio in the magnitude of distance between the song similarities and being exposed to something you could not have predicted.
When you interact with artificial intelligence, every time you’re hitting a button, a ‘like’, or a ‘skip’, on any music streaming platform, you’re training a machine. Essentially, you are telling that machine that this is what I like and based on the data it has on you, it will try and figure out what it is about that that you like compared to all these other things, i.e., what makes that song so distinct. Dr. McGraw points out a really intriguing concept that as machine learning gets better, your “experience becomes more and more like looking at a hall of mirrors, . . . and you can see yourself infinitely, right? As it gets perfected, it becomes really narcissistic, [where] you start to lose your experience of new and different data, or sounds, or people, and we see it on our Facebook feed, right? Once it figures out that I’m a middle-aged, progressive white guy, that’s all I get”. This is a concept that I am sure we are familiar with on all platforms. As soon as we type something into the Google search engine, that same thing will pop up via ads on every subsequent website you visit. Another facet of machine learning is that as we train the AI on these platforms, the total searchability of the total catalog is diminished. Spotify boasts that it “manages and shares over 82 million tracks” since its launch in 2008. (Spotify, 2022) What does that really mean in the grand scheme of things? Do we have access to all of those songs? Dr. McGraw disagrees and postulates that more or less, the more you interact with the AI, what you search for and find based on your profile, the more your visibility of the world decreases. That’s a concerning thought!
So where should we get our recommendations if utilizing streaming platforms actually diminish our chances of receiving accurate and true recommendations? YouTube, for instance, has had a history of creating diverse, even extreme results after just one click on a video. YouTube recommender systems tend to simulate an assertion where it “effectively tells them, ‘We know what question you want to ask’” and tries to finish your thought (Alfano et al. 2020, 7). Furthermore, the mere familiarity effect refers to “the fact that people tend to develop positive associations with the things, people, and concepts to which they’ve been directly exposed” (Alfano et al. 2020, 8). As a result, we are disinclined to even click on a video that disagrees with our beliefs or tastes for fear of the AI thinking we want to see it, rather than for the sole purpose of learning or exploring. Yet the YouTube community also includes albums out of print, local, folk, and traditional kinds of music that aren’t available on any major streaming service. In addition, there is a plentiful number of mixes and playlists created by fans that could populate your feed with songs that better fit your taste since they are human-made. Even scrolling through the comments is a creative forum to find out what other people think about a particular song and oftentimes, people will compare what is currently playing to another artist, or song and the user can go from there. This isn’t to say that sorting through hundreds of YouTube video comment sections will provide the best experience to find new song recommendations but it encapsulates a method that is geared towards finding similar songs based on a human ear, which has the higher potential to be successful.
Smaller, non-standard labels or curations websites like Joyful Noise Recordings are another outlet in which we may find music like. Joyful Noise claims to be “artistic facilitators for a wide variety of adventurous musicians [who] aren’t focused on any particular genre, nor … popularity. [We] value art over commerce, and we exist to empower our artists to create the most meaningful work possible”. (Joyful Recording,) Websites like these are managed, again, by a human and control the ‘Venn diagram’ of our aesthetics. Dr. McGraw stated that he’d give any new artist on this label a try, and even if he ends up only 50% of them, the fact that he trusts the website curators is significant.
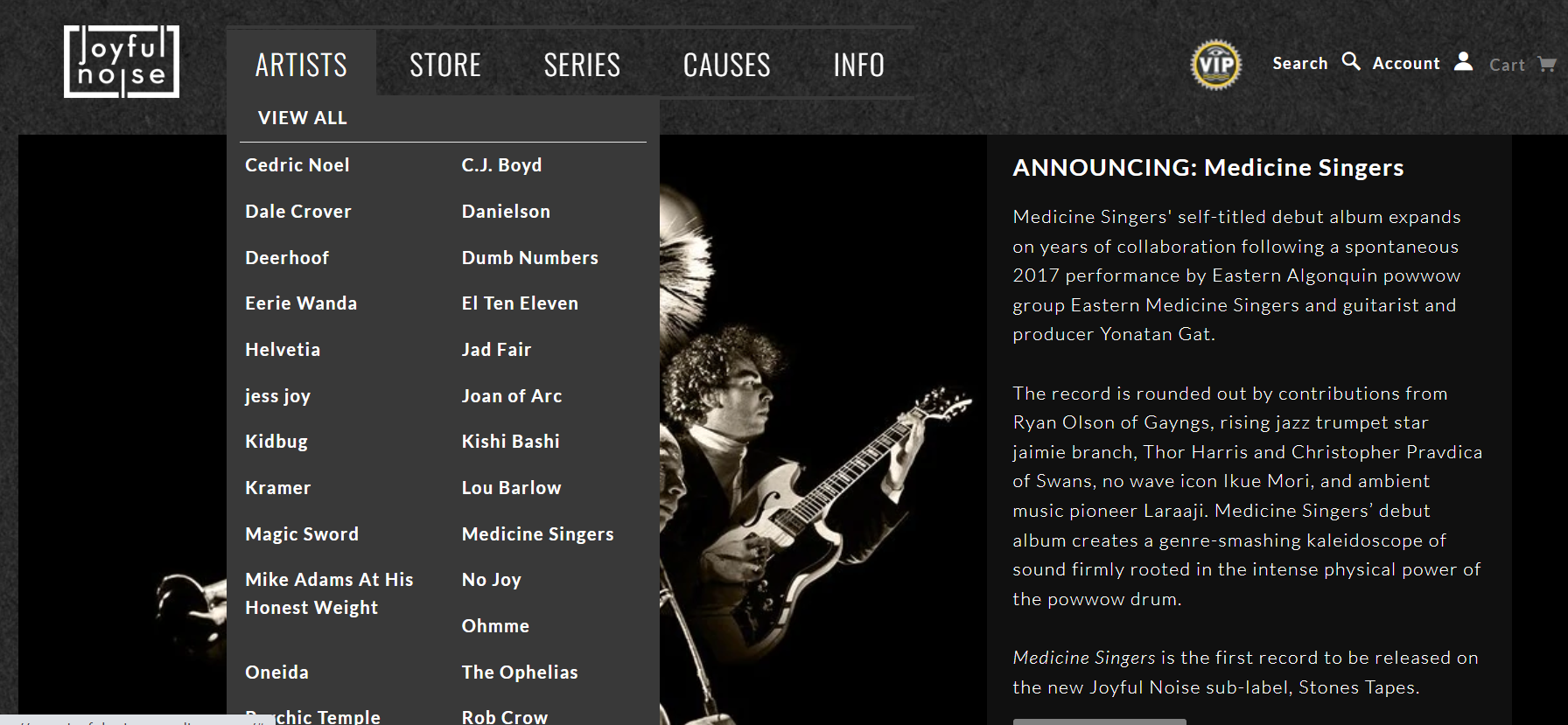
How to explore artists at https://www.joyfulnoiserecordings.com/
Moreover, Dr. McGraw reminded us that there’s a community that “rejects the model as . . . ultimately harmful for musicians”, especially for those who don’t make enough money for the social media and Spotify hustle. It takes a lot of time and an “incredible amount of effort, and what is the return? If most of your money comes from direct cash transactions and funky little gigs, then is it really worth getting into the system?”, questions Dr. McGraw. This postulation is eye-opening for listeners who may or may not only use one platform. What kind of music are we missing out on by only employing Spotify? Or Pandora? Have we thought about the artists that cannot make it onto those major labels but rather, find greater success in the local or regional scene?
Dr. McGraw has provided applicable insight on how AI within a recommender system has slowly but surely narrowed down its perception and analysis on us as listeners but in doing so, still manages to output song recommendations that we find distasteful or bad versions of what we actually like. To combat this, he suggests that we explore different tools such as YouTube mixes, boutique curation websites that share local and lesser-known artists, and go even as far as to input different songs and genres that aren’t in your usual picks to confuse the AI and generate some new suggestions. In doing so, we might discover that in which we didn’t know we liked in the first place.